Contents
背景知识 - IG 和 SmoothGrad
Expected gradients combines ideas from Integrated Gradients, SHAP, and SmoothGrad into a single expected value equation.
Integrated Gradients (IG)
使用梯度的积分来解释模型,可以解决“某特征贡献饱和时梯度为0”的问题,需要一个基线图片(与原图片多次线性插值)来做积分。
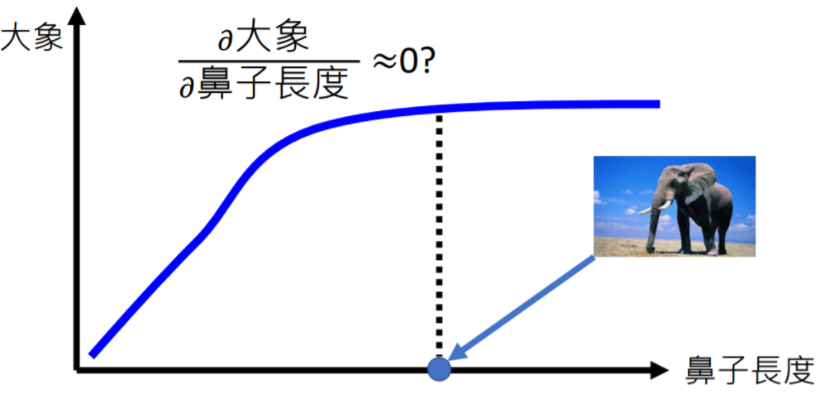

SmoothGrad
核心思想是“removing noise by adding noise”,来源于“给图片加微小扰动会造成梯度解释不稳定”的发现,解决方法是“将 n 张扰动图片的梯度平均”。